No, seriously: what the heck is expected goals (xG)?
You’ve seen it on Twitter, been confused by it on blogs and enraged by it on Match of the Day – at least if you're Jeff Stelling. But how does expected goals actually work?
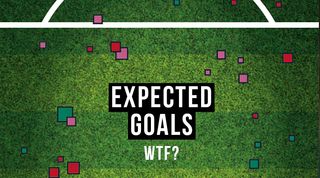
Bayern Munich probably had good reason to rue their luck after bowing out in the semi-finals of the 2015/16 Champions League against Atletico Madrid – they had lost by the finest of margins. Pep Guardiola’s side, having been beaten 1-0 in the first leg, knew they had to win the return clash in Bavaria by two clear goals.
The hosts unleashed an almighty siege on the Rojiblancos’ goal – 33 shots to Atleti’s seven, 11 of which hit the target to the visitors’ four. Yet, most tellingly, they scored two goals to their opponents’ one and were unceremoniously dumped out on the away goals rule.
The above statistics alone hinted Diego Simeone’s men might have been a touch fortunate – but a more qualitative measure suggested that their progression actually bordered on the miraculous.
"Seems I've upset the nerds"
You are talking to me about expected goals in the Champions League semi-final they’ve just lost? What an absolute load of nonsense
The next day, speaking on American sports network ESPN, Italian journalist Gabriele Marcotti mentioned in passing that, on another night, the Bundesliga giants would have achieved the result they required to reach the final – after all, their expected goals rating for the two-legged tie was 4.2 to Atleti’s 1.7.
“You are talking to me about expected goals in the Champions League semi-final they’ve just lost? What an absolute load of nonsense,” came the incredulous reply from pundit Craig Burley, the former Chelsea and Scotland midfielder clearly unimpressed with the writer’s use of the increasingly popular analytical tool.
“I expect things at Christmas from Santa Claus, but they don’t come, right? What I deal in is facts!”
Get FourFourTwo Newsletter
The best features, fun and footballing quizzes, straight to your inbox every week.
Before Marcotti could calmly expand on the finer points of expected goals – or xG, as it’s also known – the agitated Scotsman let rip again.
“Look at the results! That’s what the game is all about. Whether [or not] you or I or anybody likes it, the game is about results. That is why managers get the sack – not all this nonsense about expected goals.”
As video of the heated exchange went viral, Burley posted on Twitter: “Seems I’ve upset the nerds.”
A predictable response
Marcotti, and football’s burgeoning analytics community then took a deep breath – funnily enough, this was exactly the kind of reaction they had now come to expect. To the uninitiated, expected goals can appear like little more than an overwhelmingly complex equation. However, when you break it down, the very essence of the idea is one fans, pundits and managers have been sidestepping for decades.
“The reason I like expected goals is that it’s quite intuitive when you try to strip the math out,” says the writer and analytics expert Michael Caley, who has been exploring expected goals for a number of years. He has shared his discoveries in written articles and social media posts that have helped popularise xG among number-crunching supporters and journalists.
“Basically, it’s the idea of trying to evaluate the quality of scoring chances,” he explains. “When a pundit on television claims a team was a bit unlucky and that they could have won a game, what they’re trying to say is that the team created better scoring chances, but the goals just didn’t come.”
It may have only started appearing on Match of the Day this season (more on that later), but xG has been around for more than five years and continues to be constantly refined as more matches are played.
“Opta first came up with the concept of expected goals when one of our data scientists – Sam Green, who has since gone on to work at a Premier League club – devised an analytical model based on similar things being done in American sport,” says Duncan Alexander, Opta’s head of data editorial.
“Once the theory existed, various people in the analytics community worked on and adjusted it – making a few little tweaks to the model to try to perfect it. So there are actually several different xG models in existence, but there is only really a very slight difference with the numbers.”
Expected goals uses a whole bunch of indicators based on Opta’s on-ball event data... to determine exactly how likely it is that a particular opportunity will result in a goal
Among those to have tweaked the xG model is Caley, who originally began toying with football analytics in his spare time while studying for a PhD in the History of Religion at Harvard University. He’s therefore well placed to explain, in layman’s terms, how the whole thing works.
“Expected goals uses a whole bunch of indicators based on Opta’s on-ball event data – where on the pitch the shot had been taken from, what part of the body was used, the type of pass that had set up the chance, how quickly the move progressed down the pitch before the shot, the proximity of the opposition players, and so on – to determine exactly how likely it is that a particular opportunity will result in a goal.
“For example, if it’s a cross onto a player’s head, that’s going to have lower expected goals because those are more difficult to score from. If it’s a through-ball to feet, which is going to eliminate a number of defenders, that’s going to increase the chances of a goal. And if it's a corner-kick, there’ll be a load of defenders in the box so you’re less likely to score.
“You essentially pull all of that into one math equation that then spits out a number – expected goals – which can be tallied up over the course of a game or a season, and for a player or a team.”
Crystal Palace’s xG for their 1-0 defeat at Burnley in September, which ultimately cost Frank de Boer his briefly held job, was 1.74. Over the course of the 90 minutes, they spurned several presentable chances that on another day they would have buried. Burnley’s xG in the same match was a mere 0.43. The Clarets were evidently far more clinical.
At this stage, it's also worth making a key distinction – that between statistics and analytics.
“The thing that really irks me when I hear it is the word ‘stats’,” says Billy Beane – a man who certainly speaks with authority. Beane, as many readers will be aware, was at the heart of the data revolution in baseball during his time as general manager of the Oakland A’s.
His use of sabermetrics (“the use of objective data – what we would now call analytics – and mathematically finding a more efficient way of putting together a baseball team”) allowed the A’s to go toe-to-toe with Major League Baseball’s richest franchises, despite their own financial limitations. His tale was told in the book Moneyball and the 2011 movie of the same name. He’s also a huge football fan.
An important distinction
“Stats are results,” Beane tells FFT. “You can have the same outcome, such as a goal, from two different events but both of them can be very different in terms of how difficult they were. Take a [Lionel] Messi goal, where he has weaved through nine guys, versus a tap-in. Those goals are the same statistically, but they require two different skill sets – one was harder to score than the other.”
Expected goals may now be starting to appear in more post-match analysis alongside shots on target and the number of corners, but it doesn’t really belong in the same company. While statistics will tell you what has just happened, analytics is able to give you a much clearer idea of what could be yet to come.
“A good example I cite is Juventus in 2015/16,” explains Alexander. “After 10 league matches they had only won three times, but over the 10 games they had scored far fewer goals than you’d expect them to have based on the quality of their chances, and conceded more based on the quality of chances their opponents were creating. Their results had been much worse than their performances had suggested.
“The Turin side had scored 11 goals in those 10 games, when their xG was 19. At the other end they had leaked nine, when expected goals suggested it would usually have been five. Looking at those numbers, we expected things to regress to normal and, lo and behold, the Old Lady’s luck changed. In fact, they won their next 15 Serie A matches on the way to winning another title.”
The same method can be implemented to xG figures for an individual player. For example, a largely overlooked centre-forward who has not found the net too often may be about to start scoring for fun – and xG could help you see it coming.
“Harry Kane has consistently scored above his xG for the last three seasons,” says Alexander. “You are never going to sign a young striker on the basis of one season of similar numbers to Harry Kane, although these numbers will help you to spot players who, for whatever reason – be it some poor team-mates or a particularly rotten spell of luck – may be going under the radar.”
Caley – a Spurs fan – was able to use the model to predict Kane’s rise to goalscoring greatness before he'd even achieved the status of ‘one-season wonder’.
“I wrote an article about Kane’s shot production before he’d earned a regular place in the Tottenham line-up,” Caley tells FFT. “It outlined that, in the limited minutes he was getting for Spurs, as well as while out on loan, he had been putting up the type of numbers that looked like those of an elite forward.”
Kane’s numbers during the final months of the 2013/14 campaign – when Tim Sherwood was still in charge at White Hart Lane – were, as Caley says, “through the roof”.
It’s not inconceivable that, had a shrewd Premier League rival taken note of the statistics, been a little bit bolder and made an offer for the Tottenham rookie in the summer of 2014 when he was still very much on the fringes in N17, perhaps he would have recently netted his 100th goal in their colours instead.
But English football hasn’t always welcomed change with open arms. Just as foreign managers of the ’90s were met with some bewildered gawps when they dared suggest downing pints and gorging on steak and chips may not be the perfect preparation for elite-level athletes, those who have more recently attempted to utilise analytical models to evaluate the game have been met with, at best, a mixed response.
You are never going to sign a young striker on the basis of one season of similar numbers to Harry Kane, although these numbers will help you to spot players who may be going under the radar
Poor old Gab Marcotti certainly isn’t the first person to cite analytical data in assessing a sporting fixture, only to then be immediately shot down by sceptical naysayers.
“We weren't interested in convincing people – frankly it was to our advantage that no one was convinced,” Beane admits to FFT, speaking of his early work in baseball.
A tough sell
Despite it becoming increasingly clear that analytics has got plenty to offer, there are still doubters. When xG was made a part of Match of the Day’s graphics from the start of this Premier League campaign, suddenly it was mainstream. Within minutes of its first appearance on screen, social media was instantly awash with mentions of ‘hipsters and stat nerds’, demands for the BBC to ‘get in the sea’ and endless assertions that the numbers are ‘pointless’ and ‘bollocks’.
This was precisely why, as Match of the Day’s editor Richard Hughes explains, the programme always planned for the inclusion of expected goals to not be too intrusive.
We’ve never demanded that people use our data or claimed this stuff is going to replace humans. Expected goals is going to help football clubs make decisions and help pundits illustrate their point. It’s not going to replace the human eye
“Match of the Day attracts a lot of debate on Twitter and something new like expected goals will always divide opinion – that is why we’ve deliberately made it a pretty low-key introduction,” Hughes tells FFT.
“It's there for people who know about xG already and are keen to see it, but it’s not detracting from the experience of those who don’t.
“We’ve worked very closely with Opta over the past few seasons to integrate a lot more data into the show, and this seemed like a natural progression – something new and innovative. We have had more and more data on screen – not necessarily things that have been spoken about by the pundits, but rather support the visuals that have backed up the points they are making.”
Opta’s Alexander concurs that analytical models such as xG won’t ever replace living, breathing scouts or pundits, but merely aid them.
“We’ve never been zealots,” he says. “We’ve never demanded that people use our data or claimed this stuff is going to replace humans. Expected goals is going to help football clubs make decisions and help pundits illustrate their point. It’s not going to replace the human eye.
“Ultimately, what all these models should do is throw up a little bit of insight and then help people to form cogent arguments,” he adds.
“I would be lying if I said the pundits weren’t a tad sceptical in terms of the value it brings,” admits Hughes. “Gary Lineker, Ian Wright and Alan Shearer know quite a lot about scoring goals, and there have been variables in the model that they’ve questioned when we’ve discussed it – in particular, things such as defensive positioning and long-shot chances. The key for them is always which player has taken the shot.”
So the strikers’ union will always have their say on the performances of their brethren – regardless of the rise of xG – but what about other areas of the pitch? Will we end up having some similar conversations about defensive contributions?
“Events on the ball are what we all focus on, but there are so many other things going on that will impact what happens next,” explains Beane. “There are things that happen on a football field that aren’t being measured, so players don’t get the credit for them. For example, a defensive player, who by virtue of his ability is able to get himself into a position to alter a shot, will completely change the dynamic of the play despite never touching the ball. Eventually, that is the kind of thing you want to measure.”
The good news for Beane and the world’s best centre-backs is that an analytical way of assessing defensive contribution is in the pipeline.
“Expected goals is the first model and the one that has received the most coverage, but it’s the first in a series of hopefully quite a few we will be using,” says Alexander. "We’re also now working with ‘expected assists’, which is similar to xG, and ‘sequences’ from which you derive a team’s style of play and the pace at which they attack.
“And we’re also working on something called ‘defensive coverage’, which could be big for us because the criticism of Opta event data – and a reasonably valid one – has been that it’s a lot harder to assess defending than it is attacking.”
The future?
Defensive coverage can measure the area of defensive responsibility implied by a player’s defensive actions throughout a match – tackles, blocks, interceptions, clearances etc. So Chelsea’s all-action midfield lunatic N’Golo Kante, for instance, may cover a large area of the pitch, while a full-back in a team that’s being dominated by the opposition will likely have a smaller area.
“A good example of that from last season was when Ander Herrera marked Eden Hazard out of the game [between Manchester United and Chelsea] at Old Trafford in April,” says Alexander. “He’s nominally a central midfielder, but the Spaniard’s ‘defensive zone’ was a rough parallelogram on the edge of the right-hand side of United’s box. He was tasked with stopping Hazard, who ultimately didn’t have a single touch inside the penalty area.
“Any pundit who watched that match would certainly have spotted that Herrera performed very well, but up until now there hasn’t really been a way of illustrating that.”
That may not be music to the ears of Craig Burley, half of Twitter and anyone else who’d rather stick their fingers in their ears and pretend football’s ‘data revolution’ isn’t actually happening. But as Billy Beane puts it, “the genie’s out of the bottle now, and it’s not going back in”.
This feature originally appeared in the November 2017 issue of FourFourTwo. Subscribe!
Thank you for reading 5 articles this month* Join now for unlimited access
Enjoy your first month for just £1 / $1 / €1
*Read 5 free articles per month without a subscription
Join now for unlimited access
Try first month for just £1 / $1 / €1